本文最后更新于:2024年5月7日 下午
异常检测在工业检测等场景有重要应用,PatchCore 在 MVTec 数据集中霸榜了很久,本文介绍相关内容。
异常检测
- 异常检测是无监督学习的一种应用场景
- 通过对正常数据建模,获取对正常数据的表示模型
- 该模型在表示异常数据时会有异常的表现,以此描述异常数据
PatchCore
在异常检测算法 MVTec 数据集的 排名:
算法流程
提取特征
特征级别
- 用预训练模型 Backbone 提取图像特征
- 以 Resnet50 为例,原文放弃了局部正常特征数据较少、偏向于分类任务的深层特征,采用第 [2, 3] 层特征作为图像特征
Patch 特征
-
将图像分为 $p\times p$ 的小块提取图像的 patch特征,这个特征带有周围数据的信息
-
以每个 patch 中间位置 $h,w$ 为中心,取边长为 $p$ 的正方形区域
$$ \begin{aligned} \mathcal{N}_{p}^{(h, w)}=\{(a, b) \mid a& \in[h-\lfloor p / 2\rfloor, \ldots, h+\lfloor p / 2\rfloor] \\ b &\in[w-\lfloor p / 2\rfloor, \ldots, w+\lfloor p / 2\rfloor]\} \end{aligned} $$ -
围绕这些点计算的特征图上的点为
- 一幅图像的 Patch 特征集合为:
构建 Memory Bank
Memory Bank
-
将收集到的正常图像 Patch 特征放入 MemoryBank
$$ \mathcal{M}=\bigcup_{x_{i} \in \mathcal{X}_{N}} \mathcal{P}_{s, p}\left(\phi_{j}\left(x_{i}\right)\right) $$
Reduce memory bank
- 通过稀疏采样在尽可能保持特征表达能力的情况下,使得 MemoryBank 稀疏化(为了加快算法运行速度)
-
在确定目标Bank 特征数量后通过优化以下目标实现稀疏化
$$ \mathcal{M}_{C}^{*}=\underset{\mathcal{M}_{C} \subset \mathcal{M}}{\arg \min } \max_{m \in \mathcal{M}} \min _{n \in \mathcal{M}_{C}}\|m-n\|_{2} $$
含义为找到一个特征集合 $\mathcal {M}_ {C} $,使得 $\mathcal{M}$ 中任意特征距离 $\mathcal{M}_{C}$ 中最近的特征的距离的最大值最小
- 之后用一些选择特征的策略获取稀疏化 Memory Bank
梳理构建流程
异常检测
- 构建 Memory Bank 相当于使用正常数据进行模型训练、构建
- 之后输入待检测的图像进行异常检测,相当于 infer
infer 流程
- 提取 Patch 特征:
- 对于每个 Patch 特征选择 $\mathcal{M}_{C}$ 中距离最近的特征 $m^*$
公式 $ \arg \min \left || \mathrm{m}^{\text {test }}-\mathrm{m}\right || $ 计算的是 点 $ \mathrm{m}^{\text {test }} $ 到集合 $ \mathrm{M} $ 的距离,选择距离最远的异常值
距离最远的点为图像的异常值分数
- 文中强调为了提升算法鲁棒性,采用一个公式更新了异常值 $s^*$
我的理解为:对于特征稀疏的区域倾向于判定为异常,反之给予异常值一定的削减
每个点的异常值拼接起来即可获得图像的异常热力图
原始论文
参考资料
- Towards Total Recall in Industrial Anomaly Detection
文章链接:
https://www.zywvvd.com/notes/study/deep-learning/anomaly-detection/patchcore/patchcore/
“觉得不错的话,给点打赏吧 ୧(๑•̀⌄•́๑)૭”
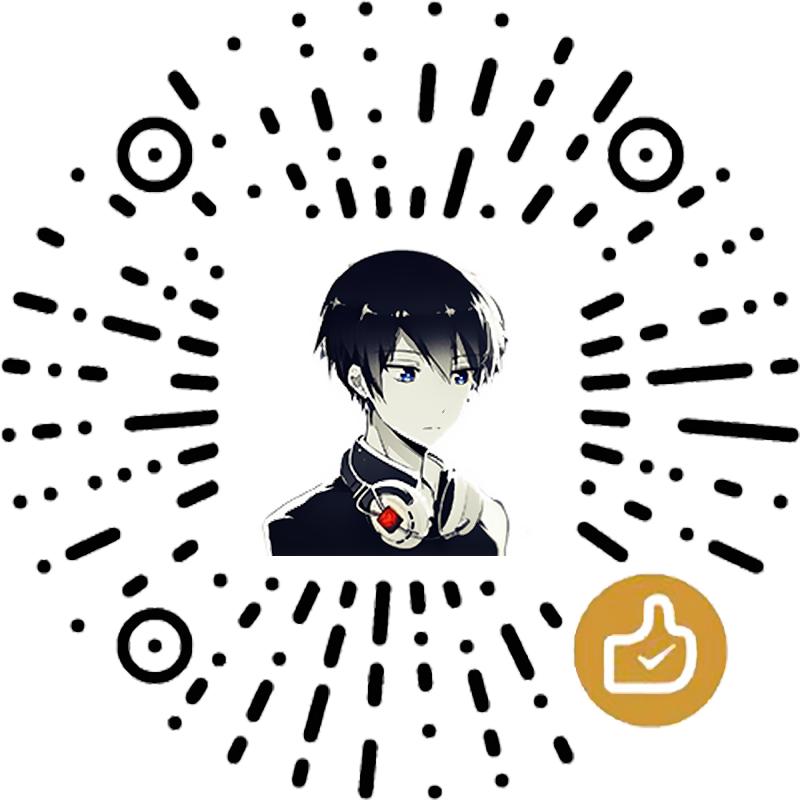
微信支付
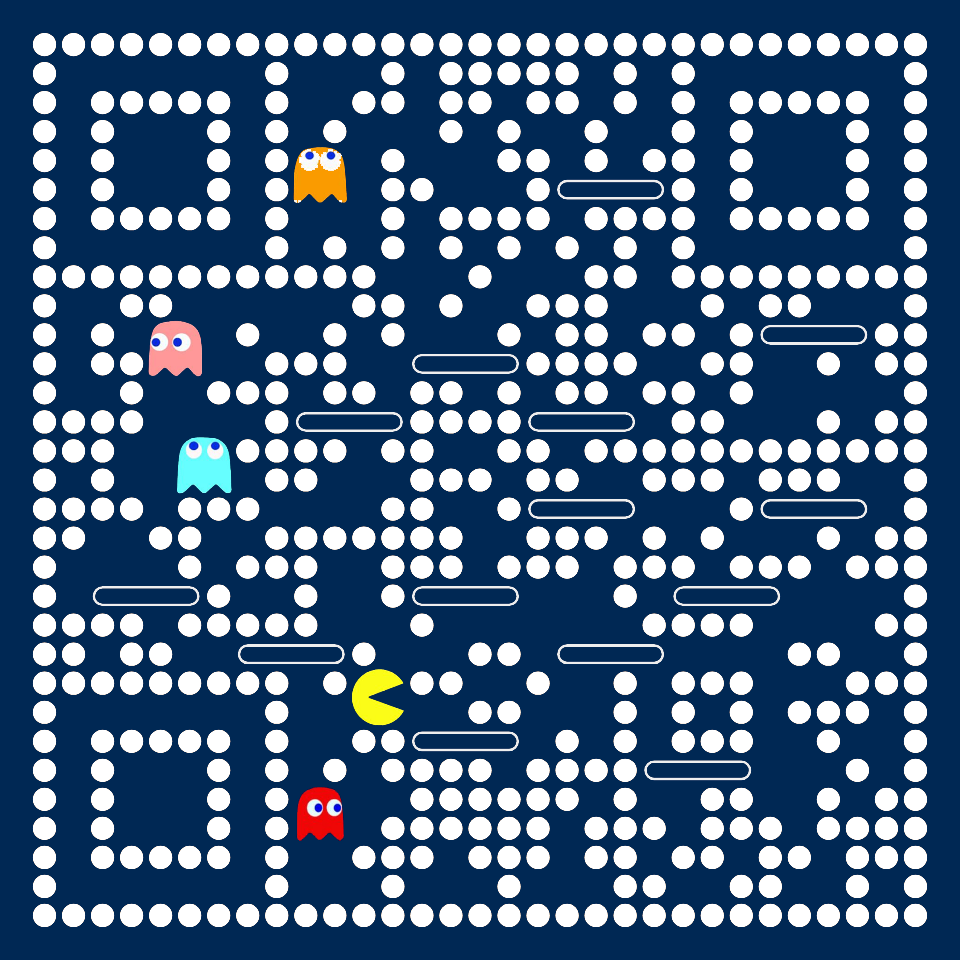
支付宝支付